Assitan Koné
Jul 1
6 Things the Experts Don’t Tell You About Machine Learning (#3 Will Blow Your Mind)
Introduction
Machine learning (ML) is often portrayed as a powerful, almost magical tool capable of solving complex problems across industries. While experts and tutorials focus on the basics of building models and the exciting potential of ML, there are crucial aspects of the process that don’t always make it into the spotlight. Here are six essential things the experts don’t always tell you about machine learning—and why understanding them is key to your success.
1. Data Quality is Everything interpretability challenges
Experts frequently emphasize the importance of data in machine learning, but they might not fully convey just how critical data quality is to the success of your project. Poor or biased data can lead to skewed results, inaccurate predictions, and flawed models. The process of data cleaning, preprocessing, and ensuring that your data is representative of the problem you're solving is time-consuming but absolutely essential.
High-quality data is the backbone of effective ML models. Investing time in data preparation can save you from costly errors down the line.
2. Model Interpretability Challenges
Building accurate models is often the primary focus, but experts don’t always highlight the challenges of model interpretability. As machine learning models become more sophisticated, they can evolve into "black boxes," making it difficult to understand how they arrive at their decisions. Explaining these complex models to stakeholders, ensuring fairness, and addressing potential biases requires careful consideration and is far from straightforward.
As you work with more complex models like deep learning networks, prioritize developing methods to interpret and explain your results to ensure transparency and trust.
3. Continuous Learning and Maintenance (This Will Blow Your Mind)
One of the most overlooked aspects of machine learning is the need for continuous learning and maintenance. Models aren’t static—they require regular updates and fine-tuning to adapt to new data, changing trends, and evolving business needs. The initial development phase is just the beginning; maintaining and evolving your models over time is equally crucial to their long-term success.
Treat your ML models as dynamic entities that need ongoing attention and updates to stay relevant and effective in a changing environment.
4. The Resource-Intensive Nature of Machine Learning
Building and optimizing machine learning models is more than just writing code. It’s a resource-intensive process that demands significant computational power, time, and infrastructure. The hardware requirements for training large-scale models, especially those involving deep learning, can be substantial. Additionally, deploying models in production introduces challenges related to scalability, latency, and cost management that are often glossed over in introductory materials.
Be prepared to invest in the necessary resources, including cloud computing and specialized hardware, to support your machine learning initiatives.
5. Human Expertise is Still Essential
While machine learning can automate many processes, human expertise remains invaluable. Effective machine learning projects often involve close collaboration between data scientists and domain experts. Domain knowledge is crucial for tasks like feature engineering, understanding model outputs, and making informed decisions that align with the specific context of the problem at hand.
Never underestimate the importance of domain expertise. The best ML solutions are developed through a synergy of data science and deep industry knowledge.
6. Ethical and Social Implications
Beyond the technical complexities, there are significant ethical and social implications tied to the deployment of machine learning models. Issues such as bias in training data, the potential for reinforcing social inequalities, and concerns around privacy and employment can be easily overlooked in the rush to innovate. These considerations are critical for developing responsible AI that aligns with societal values.
Always incorporate ethical considerations into your ML projects. Being mindful of the broader impacts of your work is essential for responsible AI development.
Conclusion
These six often-overlooked aspects of machine learning shed light on the realities of working with this powerful technology. Understanding the importance of data quality, the challenges of model interpretability, the necessity of continuous learning, the resource demands, the indispensable role of human expertise, and the ethical implications of ML is crucial for anyone serious about leveraging AI and machine learning in a meaningful way.
While these insights reveal some of the complexities behind the scenes, they don’t diminish the incredible potential of machine learning. Instead, they emphasize the need for a holistic and informed approach to maximize the benefits of ML while addressing its challenges responsibly.
#MachineLearning #Data #AIForBeginners #DeepLearning #DataScience #AI #ArtificialIntelligence #DataScienceMentorship
Want to talk about your project idea or get help finding the right one?
I offer a free 30-minute strategy call to help you:
- Get clarity on your goals
- Understand how real-world projects can unlock your next step
- See if the Accelerator is the right fit for you
Spots are limited, I work with a small number of mentees at a time.
Latest from our blog
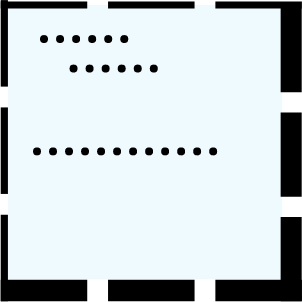
Mentorship for underrepresented talent in AI/ML
Real projects, real businesses, real transformation
Copyright ©TwaKaToz 2025