Data science is a highly sought-after career path due to its ability to extract valuable insights from large amounts of data. Despite its promising prospects, many aspiring data scientists face challenges. In this article, we explore five common difficulties and provide actionable solutions to overcome them.
Lack of Solid Foundation in Mathematics and Statistics
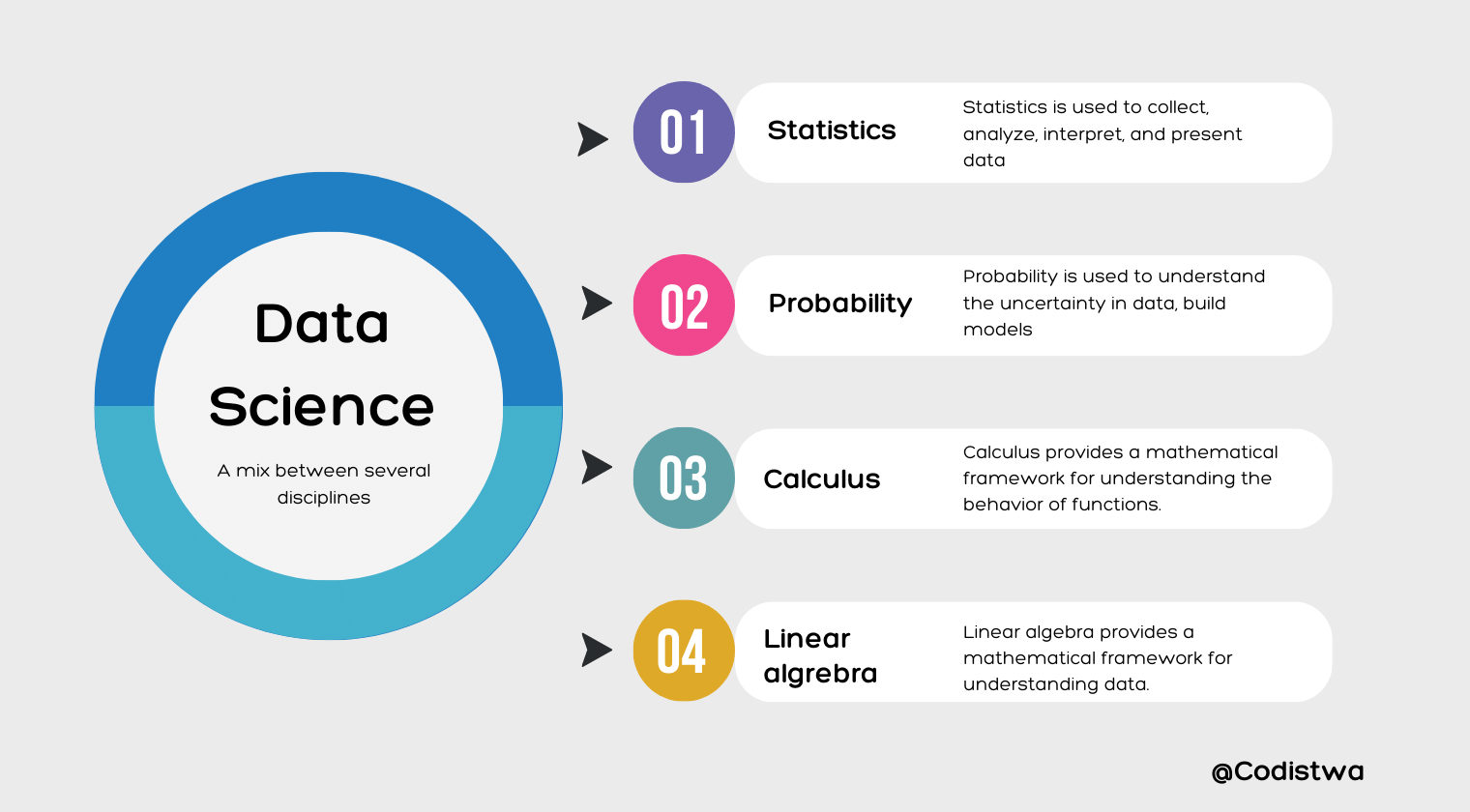
Inadequate Practical Application and Projects
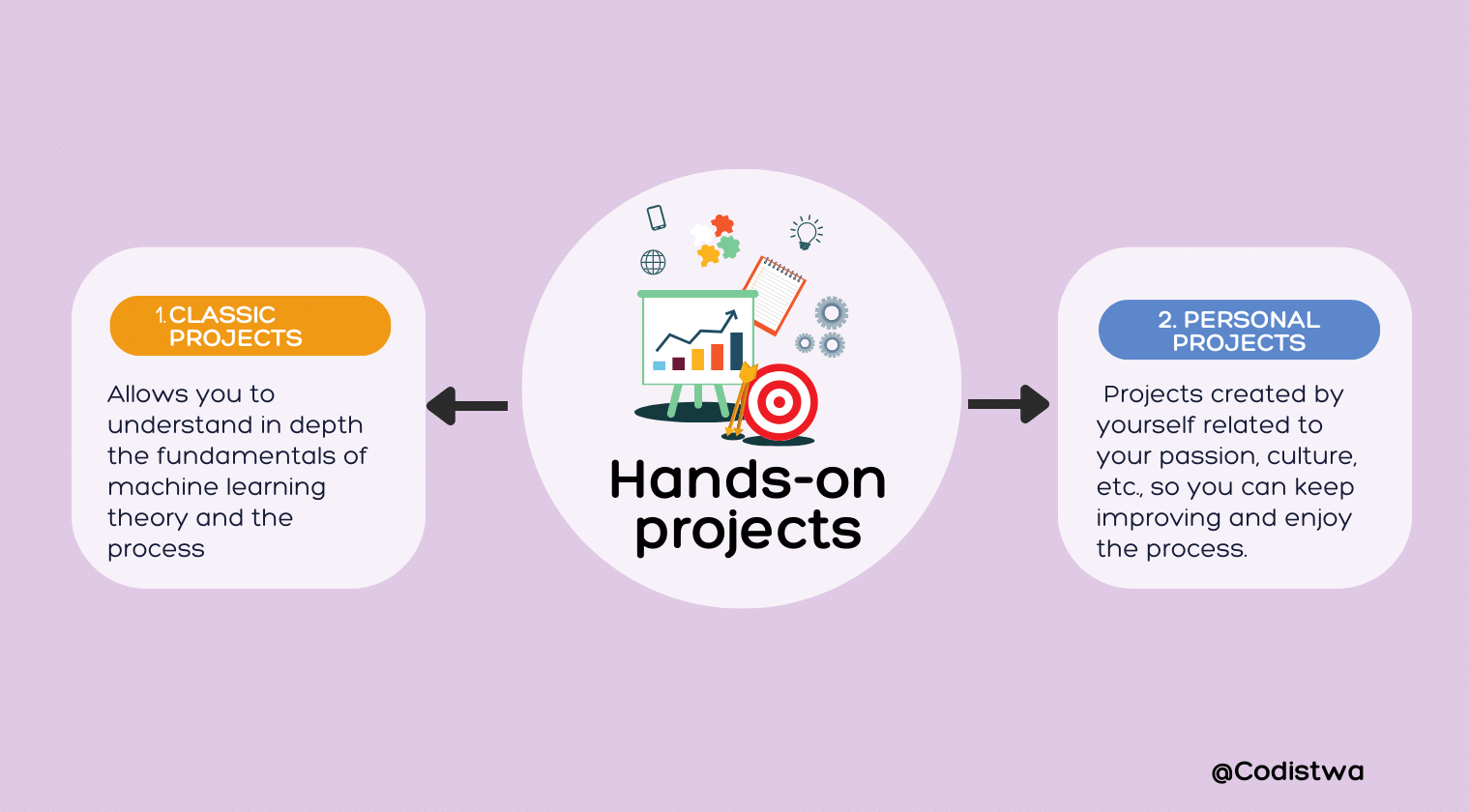
Skipping Foundational Concepts

Neglecting Soft Skills and Domain Knowledge
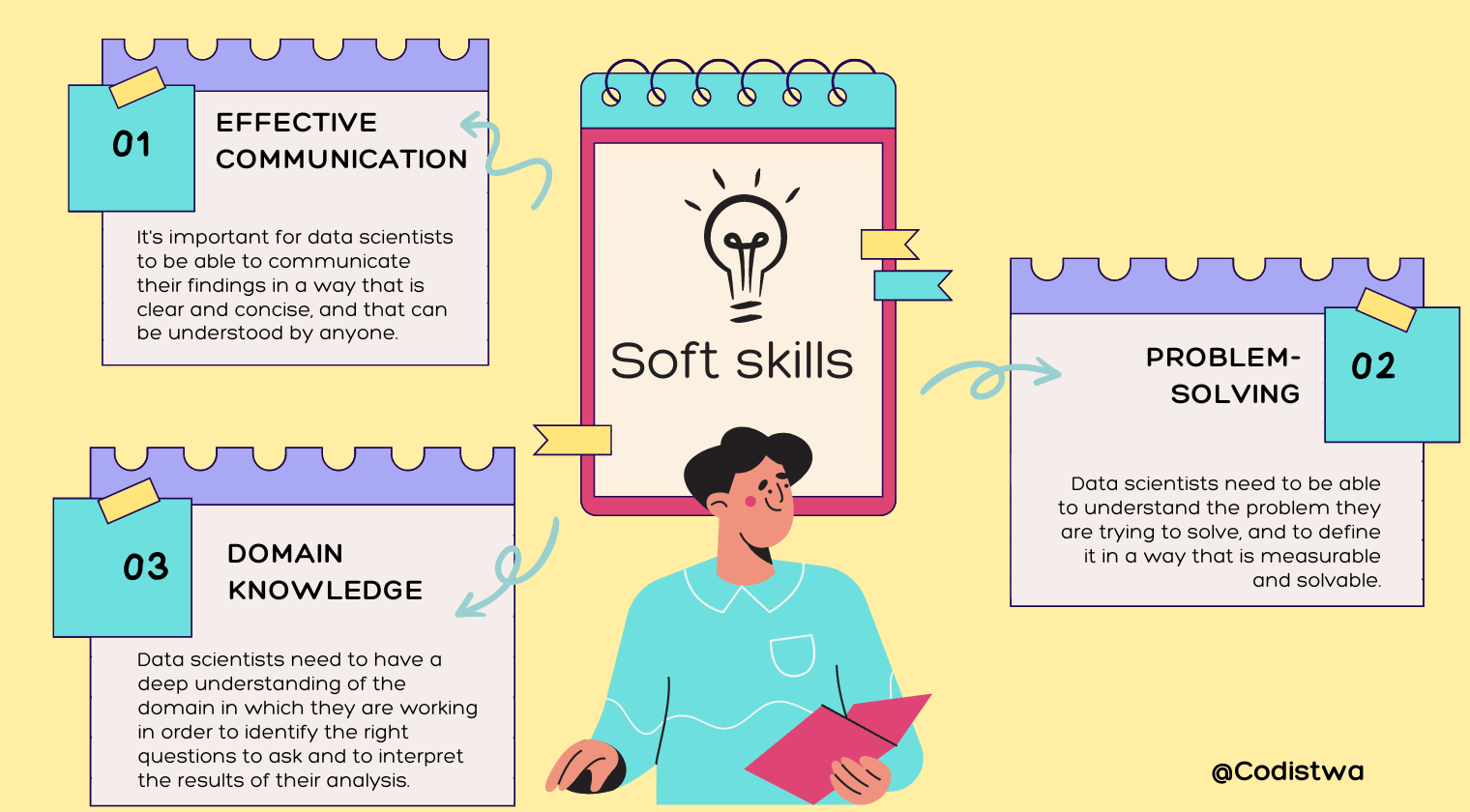
Conclusion
Write your awesome label here.
Python for Data Science Quick Start
Learn the fundamentals of Python and how to use popular Data Science libraries.
Unlock the World of Machine Learning & Deep Learning with Simple Analogies
Write your awesome label here.
Grasp Complex Concepts with Ease—Download Our Free Guide and Start Your AI/ML Journey Today!
Write your awesome label here.
FREE GUIDE: 5 Common Mistakes AI/ML Enthusiasts Make
Write your awesome label here.
Learn how to stop chasing endless tutorials and focus on what really matters: building AI/ML projects that make an impact.
Write your awesome label here.
AI & Data Science Empowerment Circle
A supportive, step-by-step paid community that will help you master data science and AI with confidence AND connect your learning to your passions, culture, and expertise—making complex concepts relatable and actionable.
Write your awesome label here.
Overcome Imposter Syndrome and Build Unshakable Confidence in Just 5 Days
Latest from our blog
Sign up. Be inspired. Code.
Get a FREE Machine Learning Roadmap!
Write your awesome label here.